Operational Artificial Intelligence: How To Bring AI To Life In Your Enterprise
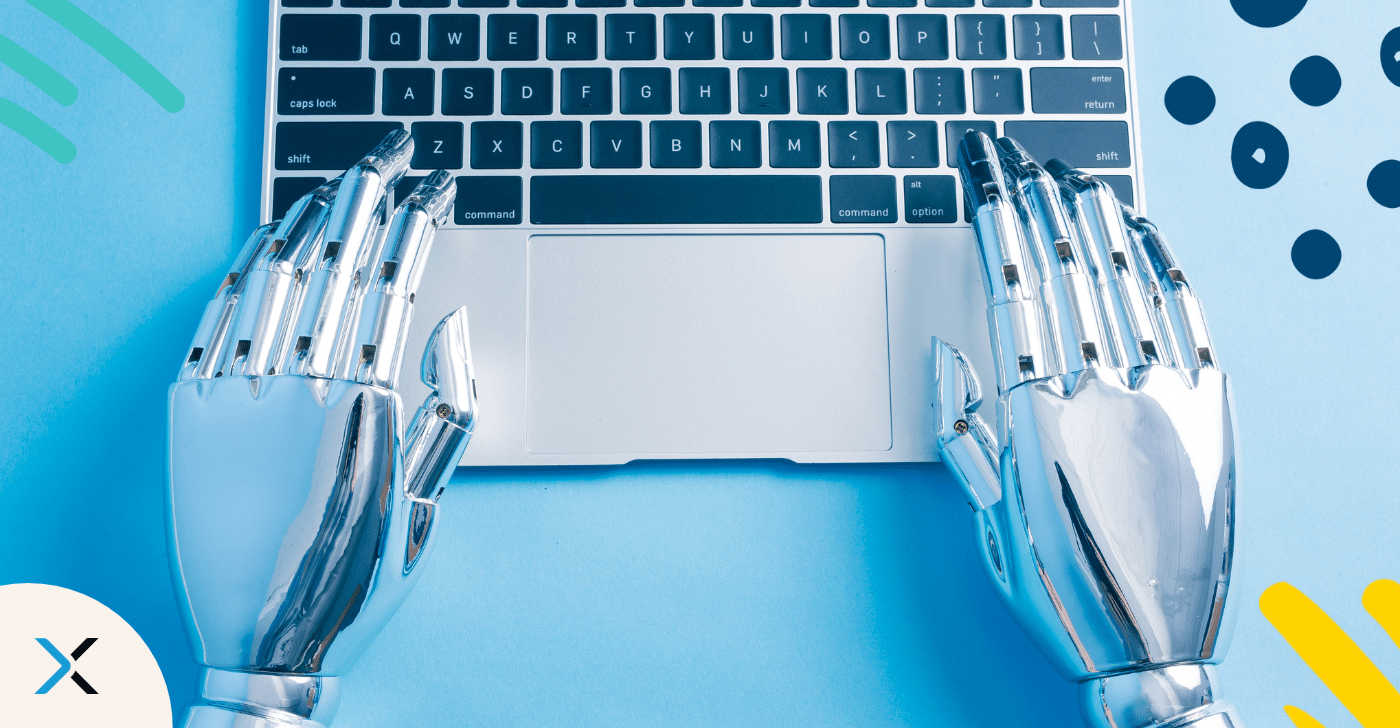
AI is everywhere — powering your recommendations, automating tasks, and writing enterprise content. But here’s the catch: Having an AI model is one thing; making it actually work at scale in your enterprise is a whole different task.
To make AI truly operational, you need a strategy that adapts to enterprise applications and stays compliant with regulations. The goal here’s to transform AI tools from ideas and possibilities into a core component of your real world business operations. Welcome to operational AI!
Whether you’re generating content, checking for compliance, or streamlining the content supply chain, AI only delivers value when it’s reliable, scalable, and governable. So, how do you make that happen? Let’s start with the fundamentals of AI operationalization.
What’s AI operationalization?
So you’ve got a new AI tool. Great! But now what? The real challenge isn’t just getting AI up and running — it’s making sure it actually works at scale, efficiently. And in a way that doesn’t land your company in legal hot water. That’s what AI operationalization is about.
AI operationalization (often called AI Ops) is the process of moving AI from experimental pilots to fully integrated, business-critical systems. It’s important that AI not only gets deployed but also actively delivers value — reliably, securely, and at scale. Many businesses assume that once they train a model, it’s ready to go. In reality, without a solid operationalization strategy, AI initiatives quickly become expensive, inefficient, and even risky.
Why enterprises need to operationalize AI
Here’s why AI operationalization is essential:
Scalability: AI needs to grow with your business
We must build AI to scale. This means it can process large volumes of data, integrate with existing systems, and adapt to increasing demands without breaking down or taking unethical shortcuts.
Governance: AI needs guardrails
With regulations like GDPR, the EU AI Act, and ethical AI standards becoming stricter, businesses must focus on compliance, transparency, and fairness. AI operationalization must include AI guardrails for your content standards so you don’t end up publishing non-compliant, off-brand content.
Efficiency: AI should save costs, not create bottlenecks
An AI tool that takes too long to process data or requires constant manual intervention is a liability, not an asset. Operationalization makes sure that AI is optimized for cost-effectiveness, automation, and long-term sustainability — so your investment actually pays off.
Without proper operationalization, AI remains an experiment. One that drains resources without delivering results. True AI success comes when models are scalable, well-governed, and efficient, powering real business impact.
Why scaling generative AI is a challenge
While many companies are eager to integrate AI into their workflows, scaling generative AI beyond small pilots to full enterprise deployment is far from simple.
As organizations adopt AI-generated content, they quickly realize that operationalizing and scaling it isn’t just about adding more computing power. It’s about supporting consistency, governance, and seamless integration into existing business processes. Without the right approach, AI becomes more of a liability than an asset — leading to inconsistent brand messaging and compliance risks.
The core challenges of scaling generative AI include:
Content quality
While generative AI can produce impressive results, it can also be unpredictable. Enterprises need mechanisms to maintain brand voice, editorial accuracy, and alignment to writing standards.
Governance and compliance
AI-generated content must follow industry regulations, copyright laws, and ethical guidelines. Without strict governance, businesses risk unclear information, biased outputs, and legal consequences.
Integration with existing systems
AI doesn’t work in a vacuum. It must seamlessly connect with CRM platforms, content management systems, and customer support tools. Meaning that it improves workflows rather than creating bottlenecks.
If you want to scale generative AI successfully, you need an operational framework that prioritizes reliability, efficiency, and compliance. This means implementing automated quality checks, clear content governance, and scalable infrastructure to support AI-powered content creation at an enterprise level.
Key steps to successful AI operationalization
The future of generative AI in business isn’t just about generating more content — it’s about doing it smarter, safer, and at scale. It requires a strategic approach to make sure AI is scalable, efficient, and aligned with business goals. Here are the essential steps enterprises must take to reach business goals with the support of AI.
Establishing strong AI governance
AI governance is the foundation of responsible AI deployment. Without it, businesses risk inconsistent outputs, ethical pitfalls, and regulatory violations. A strong governance framework should include:
Framework component | What it should do |
Clear AI policies | Define how teams train, monitor, and update AI models to align with corporate standards and ethical guidelines. |
Explainability and transparency | Make sure you can trace AI-generated content back to its sources and decisions can be explained. |
Compliance checks | Implement safeguards to identify and mitigate compliance issues in AI outputs. |
Role-based AI oversight | Assign responsibilities for AI performance, compliance, and improvement across teams. |
By putting these guardrails in place, businesses can scale AI with confidence. After all, businesses are responsible for the AI-generated content that AI tools create. Especially to make sure that it falls within legal, regulatory, and ethical boundaries.
Managing AI risks and compliance challenges
Scaling AI introduces a new level of complexity when it comes to regulations, data security, and ethical considerations. Enterprises must proactively address risks by:
- Enforcing compliance with industry regulations (for example, GDPR, CCPA, AI Act) to avoid legal repercussions.
- Implementing continuous monitoring and auditing to track AI performance and highlight potential risks.
- Safeguarding data privacy by controlling what data AI models use and encouraging proper anonymization practices.
- Setting up AI risk assessments to evaluate potential content inaccuracies, brand inconsistencies, and copyright issues.
A structured approach to compliance means that AI remains a strategic advantage rather than a liability.
Building AI into the content supply chain
For AI to be truly operational, it must integrate seamlessly into existing workflows. This means embedding AI into the content supply chain — from ideation to distribution — without disrupting established processes. Fundamental strategies include:
- Automating content creation with human oversight. Use AI to generate drafts and align to writing guidelines, while editors refine and approve content.
- Connecting AI tools with CMS, CRM, and analytics platforms. You need to publish and track your AI-generated content easily.
- Standardizing AI content review. Implement AI-powered quality checks to enforce brand voice, compliance guidance, and tone consistency.
- Optimizing AI for different content formats. Whether it’s marketing copy, support documentation, or reports, AI must adapt to different needs.
When AI becomes an inherent part of the content lifecycle, businesses unlock massive efficiency gains without sacrificing quality.
Driving user adoption and AI literacy
Even the most powerful AI models are useless if employees don’t know how to use them effectively. Fueling AI adoption and literacy leads to teams feeling confident using AI as a tool rather than fearing it as a replacement. Key steps include:
- Providing AI training programs to educate employees on best practices and limitations.
- Encouraging AI experimentation through pilot projects and hands-on workshops.
- Defining AI-human collaboration. Clarify where AI should assist vs. where human expertise is essential.
- Offering AI usage guidelines to help teams apply AI effectively while maintaining brand and regulatory standards.
A culture of AI empowerment helps businesses to scale AI adoption without resistance, leading to long-term success.
AI operationalization isn’t just about launching AI — it’s about keeping it running smoothly at scale. By implementing strong governance, managing risks, embedding AI into workflows, and fostering AI literacy, you ensure AI delivers transformative results.
The next step? Turning these strategies into action!
Learn more about scaling generative AI in 2025
Scaling generative AI is a must for businesses looking to stay competitive in an AI-powered world. But as we’ve seen, successful AI operationalization requires more than just haphazard adoption. It’s about scalability, governance, efficiency, and integration into real-world workflows. Companies that prioritize AI ops will unlock new levels of productivity, consistency, and compliance — while those that don’t risk falling behind.
So, how can your enterprise turn AI into a scalable, sustainable asset in 2025?
Watch our exclusive webinar: Scaling Success: Operationalizing Generative AI for 2025. You’ll gain expert insights, real-world examples, and practical strategies to navigate AI operationalization with confidence.
Are you ready to create more content faster?
Schedule a demo to see how content governance and AI guardrails will drastically improve content quality, compliance, and efficiency.
The Acrolinx Team